Chapter 3 A Bayesian data analysis workflow
3.1 Bayesian inference summarised
3.1.1 Motivation for a Bayesian approach
Bayesian statistics are mentioned in the Annex B of the ASHRAE Guideline 14, after it has been observed that standard approaches make it difficult to estimate the savings uncertainty when complex models are required in a measurement and verification worflow:
“Savings uncertainty can only be determined exactly when energy use is a linear function of some independent variable(s). For more complicated models of energy use, such as changepoint models, and for data with serially autocorrelated errors, approximate formulas must be used. These approximations provide reasonable accuracy when compared with simulated data, but in general it is difficult to determine their accuracy in any given situation. One alternative method for determining savings uncertainty to any desired degree of accuracy is to use a Bayesian approach.”
Still on the topic of measurement and verification, and the estimation of savings uncertainty, several advantages and drawbacks of Bayesian approaches are described by (Carstens, Xia, and Yadavalli (2018)). Advantages include:
- Because Bayesian models are probabilistic, uncertainty is automatically and exactly quantified. Confidence intervals can be interpreted in the way most people understand them: degrees of belief about the value of the parameter.
- Bayesian models are more universal and flexible than standard methods. Models are also modular and can be designed to suit the problem. For example, it is no different to create terms for serial correlation, or heteroscedasticity (non-constant variance) than it is to specify an ordinary linear model.
- The Bayesian approach allows for the incorporation of prior information where appropriate.
- When the savings need to be calculated for “normalised conditions”, for example, a “typical meteorological year”, rather than the conditions during the post-retrofit monitoring period, it is not possible to quantify uncertainty using current methods. However, (Shonder and Im (2012)) have shown that it can be naturally and easily quantified using the Bayesian approach.
The first two points above are the most relevant to a data analyst: any arbitrary model structure can be defined to explain the data, and the exact same set of formulas can then be used to obtain any uncertainty after the models have been fitted.
3.1.2 General Bayesian principles
A Bayesian model is defined by two components:
- An observational model \(p\left(y|\theta\right)\), or likelihood function, which describes the relationship between the data \(y\) and the model parameters \(\theta\).
- A prior model \(p(\theta)\) which encodes eventual assumptions regarding model parameters, independently of the observed data. Specifying prior densities is not mandatory.
The target of Bayesian inference is the estimation of the posterior density \(p\left(\theta|y\right)\), i.e. the probability distribution of the parameters conditioned on the observed data. As a consequence of Bayes’ rule, the posterior is proportional to the product of the two previous densities:
\[\begin{equation} p(\theta|y) \propto p(y|\theta) p(\theta) \tag{3.1} \end{equation}\]
This formula can be interpreted as follows: the posterior density is a compromise between assumptions and evidence brought by data. The prior can be “strong” or “weak”, to reflect for a more or less confident prior knowledge. The posterior will stray away from the prior as more data is introduced.
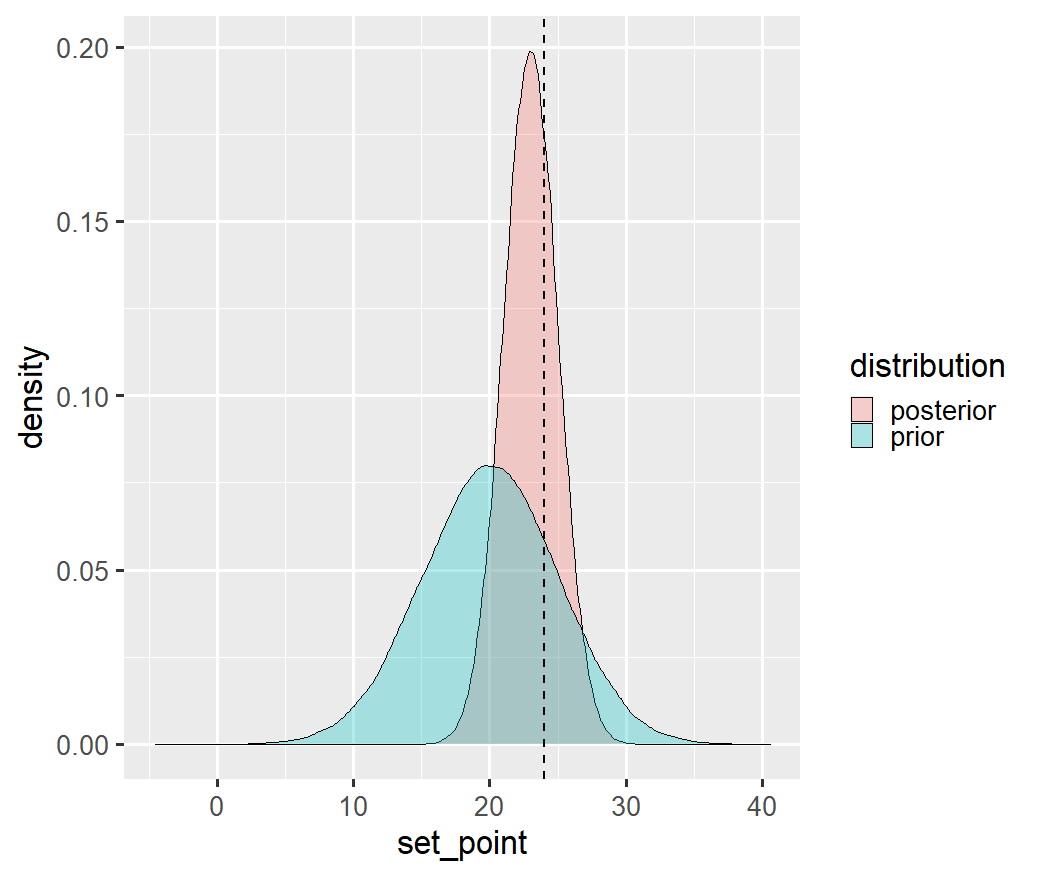
Figure 3.1: Example of estimating a set point temperature after assuming a Normal prior distribution centred around 20°C. The dashed line is the point estimate which would have been obtained if only the data had been considered. The posterior distribution can be seen as a “refinement” of the prior, given the evidence of the data.
In general, information from the posterior distribution is represented by summary statistics such as the mean, variance or credible intervals, which can be used to inform decisions and are easier to interpret than the full posterior distribution. Most of these summary statistics take the form of posterior expectation values of certain functions, \(f(\theta)\),
\[\begin{equation} \label{posterior_expectation} \mathbb{E}[f(\theta)] = \int p(\theta|y) f(\theta) \mathrm{d} \theta \tag{3.2} \end{equation}\]
More sophisticated questions are answered with expectation values of custom functions \(f(\theta)\). One common example is the posterior predictive distribution: in many applications, one is not only interested in estimating parameter values, but also the predictions \(\tilde{y}\) of the observable during a new period. The distribution of \(\tilde{y}\) conditioned on the observed data \(y\) is called the posterior predictive distribution:
\[\begin{equation} p\left(\tilde{y}|y\right) = \int p\left(\tilde{y}|\theta\right) p\left(\theta|y\right) \mathrm{d}\theta \tag{3.3} \end{equation}\]
The posterior predictive distribution is an average of the model predictions over the posterior distribution of \(\theta\). This formula is equivalent to the concept of using a trained model for prediction.
Apart from the possibility to define prior distributions, the main specificity of Bayesian analysis is the fact that all variables are encoded as probability densities. The two main results, the parameter posterior \(p(\theta|y)\) and the posterior prediction \(p\left(\tilde{y}|y\right)\), are not only point estimates but complete distributions which include a full description of their uncertainty.
3.2 Workflow for one model
3.2.1 Overview
As was mentioned in Sec. 1.2.4, inverse problems are all but trivial. It is possible that the available data is simply insufficient to bring useful inferences, but that we still try to train an unsuitable model with it. Statistical analysts need the right tools to guide model selection and training, and to warn them when there is a risk of biased inferences and predictions.
This chapter is an attempt to summarize the essential points of a Bayesian workflow from a building energy perspective. Frequentist inference is also mentioned, but as a particular case of Bayesian inference.
There is a very rich literature on the proper workflow for statistical inference, including the most cited book in this report (Gelman et al. (2013)) and extensive online tutorials. Gelman divides the process of Bayesian data analysis into three steps:
- Setting up a full probability model;
- Conditioning on observed data (learning);
- Evaluating the fit of the model and the implications of the resulting posterior (checking and validation).
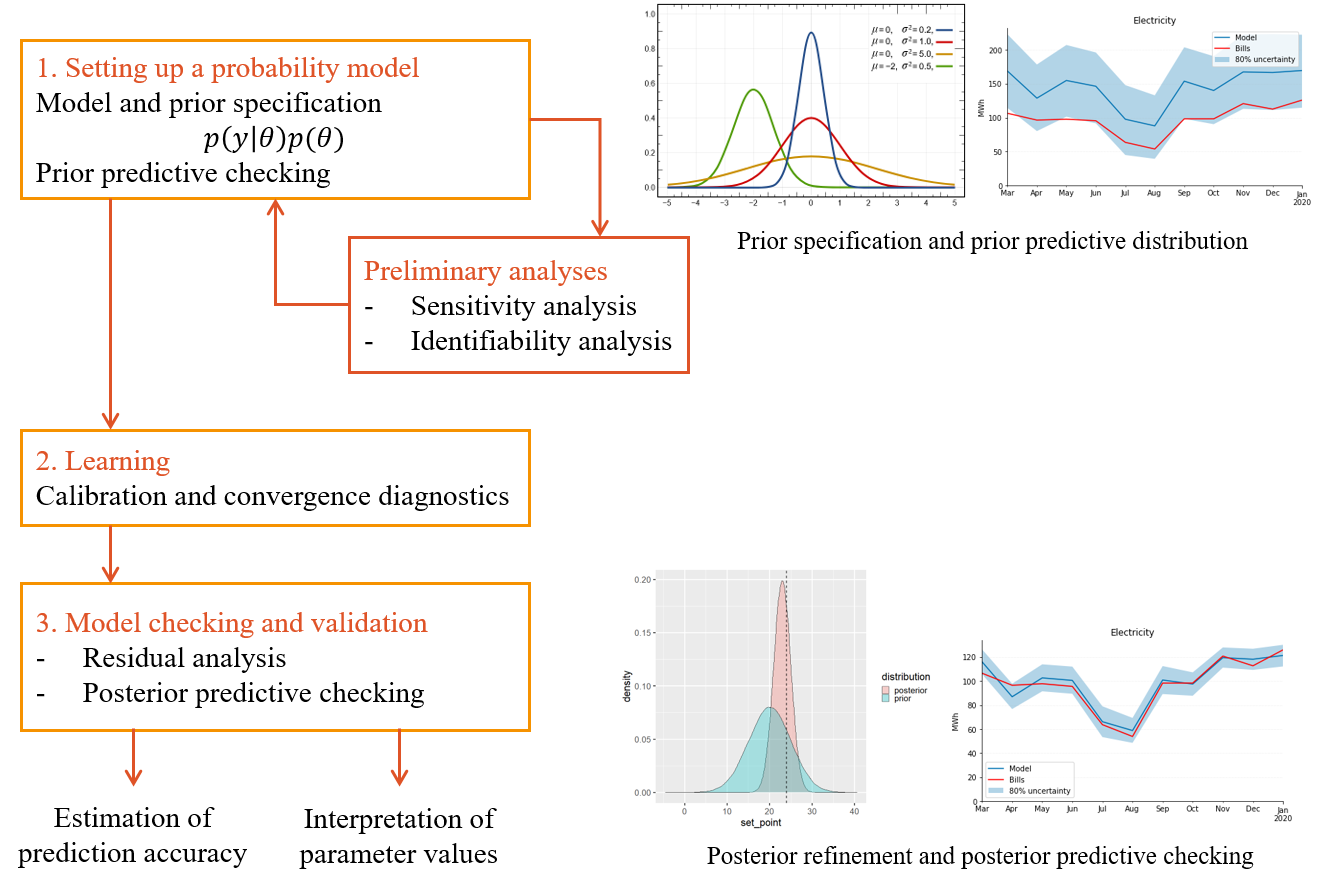
Figure 3.2: A workflow for the proper specification and training of one model. Most of the workflow is similar for frequentist and Bayesian inference.
Fig. 3.2 gives an overview of these three steps, which will be detailed in the present section. An additional step of preliminary analyses may be included to Gelman’s formulation of the model definition: sensitivity analysis and identifiability analysis are two categories of methods which may prevent over-parameterisation and ill-posedness of the inverse problem. Their outcome may incite to reformulate the probability model.
This process concerns the training of a single model. An analyst however rarely attempts to analyze data with a single model. A simple model will provide biased inferences and predictions if its structure is too simple to represent the real system. In a complex model with many degrees of freedom, the unicity of the solution to the inverse problem is not guaranteed, leading to non-robust inferences and overfitting. All statistical learning lectures therefore come with guidelines for model checking, assessment and selection: this will be explained in Sec. 3.3.
3.2.2 Step 1: model specification
The first step into building our model is a conceptual analysis of the system and the available data. The first question is to decide what we want to learn from the data, and is related to the choice of measurement and modelling boundaries mentioned in Sec. 2.2, and the choice of model structure mentioned in Sec. 2.3: which of the measurements is the dependent variable \(y\), which are the relevant explanatory variables \(x\), and how will the model parameters \(\theta\) be defined.
In Sec. 1.3, we have roughly separated the analyses in two categories: prediction and inference.
- If the main goal is prediction (of energy use, occupation, ambient variables…) then the choice of the dependent variable is straightforward, but there is a lot of freedom in the choice of explanatory variables and model structure \(p(y|\theta)\).
- If the main goal is inference (of some energy performance index such as HTC) then the choice of modelling boundaries \(x\) and \(y\) is not trivial, but the parameterisation of the model will be constrained so that \(\theta\) can be related to the inference goal.
In both situations, the model definition is greatly impacted by the time resolution and length of the dataset. Higher time resolutions (under an hour) enable the choice of dynamical models, which can encode more inferential information but imply a more complex development. Longer datasets (several months) enable the aggregation of data over longer resolutions and observations covering different weather conditions.
The next step is the development of the model: the translation of the conceptual narrative of the system into formal mathematical descriptions. The target is to formulate the entire system into probabilities that our fitting method can work with. In the case of simple regression models, the observational model may be summarized by a single likelihood function \(p(y|\theta)\), eventually conditioned on explanatory variables.
If the practitioner wishes to use a regression model to explain the relationship between the parameters and the data, doing so in a Bayesian framework is very similar to the usual (frequentist) framework. As an example, a Bayesian model for linear regression with three parameters \((\theta_0,\theta_1,\theta_2)\) and two explanatory variables \((X_1,X_2)\) may read: \[\begin{align} p(y|\theta,X) & = N\left(\theta_0 + \theta_1 X_1 + \theta_2 X_2, \sigma\right) \tag{3.4} \\ p(\theta_i) & = \Gamma(\alpha_i, \beta_i) \tag{3.5} \end{align}\]
This means that \(y\) follows a Normal distribution whose expectation is a linear function of \(\theta\) and \(X\), with standard deviation \(\sigma\) (the measurement error). The second equation is the prior model: in this example, each parameter is assigned a Gamma prior distribution parameterised by a shape \(\alpha\) and a scale \(\beta\). Other model structures can be formulated similarly: change-point models, polynomials, models with categorical variables… Bayesian modelling however allows for much more flexibility:
- Other distributions than the Normal distribution can be used in the observational model;
- Hierarchical modelling is possible: parameters can be assigned a prior distribution with parameters which have their own (hyper)prior distribution;
- Heteroscedasticity can be encoded by assuming a relationship between the error term and explanatory variables, etc.
More complex models with latent variables have separate expressions for the respective conditional probabilities of the observations \(y\), latent variables \(z\) and parameters \(\theta\). In this case, there is a likelihood function \(p(y,z|\theta)\) and a marginal likelihood function \(p(y|\theta)\) so that: \[\begin{equation} p(y|\theta) = \int p(y,z|\theta) \mathrm{d}z \tag{3.6} \end{equation}\]
Other applications, such as the IPMVP option D, rely on the use of calibrated building energy simulation (BES) models. These models are described by a much larger number of parameters and equations that the simple regression models typically used for other IPMVP options. In this context, it is not feasible to fully describe BES models in the form of a simple likelihood function \(p(y|\theta)\). In order to apply Bayesian uncertainty analysis to a BES model, it is possible to first approximate it with a Gaussian process (GP) model emulator. This process is denoted Bayesian calibration and was based on the seminal work of Kennedy and O’Hagan (Kennedy and O’Hagan (2001)). As opposed to the manual adjustment of building energy model parameters, Bayesian calibration explicitly quantifies uncertainties in calibration parameters, discrepancies between model predictions and observed values, as well as observation errors (Chong and Menberg (2018)).
3.2.3 Prior predictive checking
The full probability model is the formalization of many assumptions regarding the data-generating process. In theory, the model can be formulated only based on domain expertise, regardless of the data. In practice, a model which is inconsistent with the data has little chance to yield informative inferences after training. The prior predictive distribution, or marginal distribution of observations \(p(y)\), is a way to check for the consistency of our expertise.
\[\begin{equation} p\left(y\right) = \int p\left(y|\theta\right) p\left(\theta\right) \mathrm{d}\theta \tag{3.7} \end{equation}\]
Basically, computing this distribution is equivalent to running a few simulations of a numerical model before its training, with some assumed values of the parameters. In Bayesian terms, we first draw a finite number of parameter vectors \(\tilde{\theta}^{(m)}\) from the prior distribution, and use each of them to compute a model output \(\tilde{y}^{(m)}\):
\[\begin{align} \tilde{\theta}^{(m)} & \sim p(\theta) \tag{3.8} \\ \tilde{y}^{(m)} & \sim p(y|\tilde{\theta}^{(m)}) \tag{3.9} \end{align}\]
This set of model outputs approximates the prior probability distribution. If large inconsistencies can be spotted between this distribution and measurements, we can adjust some assumptions regarding the prior definition or the structure of the observational model. An example of prior predictive checking is shown on the top right of Fig. 3.2, which suggests a model structures which does not contradict the observations.
3.2.4 Step 2: computation with Markov Chain Monte Carlo
Except in a few convenient situations, the posterior distribution is not analytically tractable. In practice, rather than finding an exact solution for it, it is estimated by approximate methods. The most popular option for approximate posterior inference are Markov Chain Monte Carlo (MCMC) sampling methods. When it is not possible or not computationally efficient to sample directly from the posterior distribution, Markov Chain simulation is used to stochastically explore the typical set, i.e. the regions of parameter space which have a significant contribution to the desired expectations. Markov chains used in MCMC methods are designed so that their stationary distribution is the posterior distribution. If the chain is long enough, the state history of the chain provides samples from the typical set \(\left(\theta^{(1)},...,\theta^{(S)}\right)\) \[\begin{equation} \theta^{(s)} \sim p(\theta | y) \tag{3.10} \end{equation}\] where each draw \(\theta^{(s)}\) contains a value for each of the parameters of the model.
Commonly used MCMC algorithms, such as Metropolis-Hastings or Gibbs sampler, are inefficient in high-dimension because of their random walk behavior. As the dimension increases, the typical set becomes narrower and good guesses become rarer; the Markov chain may get stuck for a potentially long time. Hamiltonian Monte Carlo (HMC) algorithm alleviates this issue by exploiting information about the geometry of the typical set (Betancourt (2017)). The HMC algorithm supresses the random walk behavior by borrowing an idea from physics. Metaphorically, the vector of parameters represents the position of a frictionless particle which follows a physical path determined by the curvature of the posterior distribution. Furthermore, the gradient of the logarithm of the posterior distribution guides the Markov chain along regions of high probability mass which provides an efficient exploration of the typical set.
HMC is a state-of-the-art algorithm for Bayesian inference and is made available by software libraries such as the STAN language. Applications of HMC to the calibration of building energy models include whole building simulation (Chong and Menberg (2018)) and state-space models (Lundström and Akander (2020)).
Posterior expectation values can be accurately estimated by Monte Carlo estimator. Based on exact independent random samples from the posterior distribution \(\left(\theta^{(1)},...,\theta^{(S)}\right) \sim p(\theta | y)\), the expectation of any function \(f(\theta)\) can be estimated with \[\begin{equation} \mathbb{E}[f(\theta)] \approx \frac{1}{N} \sum_{n=1}^{N} f(\theta)^{(n)} \tag{3.11} \end{equation}\]
Markov Chain Monte Carlo estimators converge to the true expectation values as the number of draws approaches infinity. In practice, diagnostics must be applied to check that the estimator follows the central limit theorem, which ensures that the estimator is unbiased after a finite number of draws. For that purpose it is first recommended to compute the (split-)\(\hat{R}\) statistic, or Gelman-Rubin statistic, with multiple chains initialized at different initial positions and split into two halves (Gelman et al. (2013)). The \(\hat{R}\) statistic measures for each scalar parameter, \(\theta\), the ratio of samples variance within each chain \(W\) to the sample variance of all combined chains \(B\),
\[\begin{equation} \hat{R} = \sqrt{\frac{1}{W} \left(\frac{N-1}{N}W + \frac{1}{N}B \right)} \tag{3.12} \end{equation}\]
where \(N\) is the number of samples. If the chains have not converged, \(W\) will underestimate the variance, since the individual chains have not had time to range all over the stationary distribution, and \(B\) will overestimate the variance, since the starting positions were chosen to be overdispersed.
Another important convergence diagnostics tool is the effective sample size (ESS), defined as:
\[\begin{equation} \text{ESS} = \frac{N}{1 + 2 \sum_{l=1}^{\infty} \rho_l} \tag{3.13} \end{equation}\]
with \(\rho_l\) the lag-\(l\) autocorrelation of a function \(f\) over the history of the Markov chain. The effective sample size is an estimate of the number of independent samples from the posterior distribution.
The diagnostic tools introduced in this section provide a principled workflow for reliable Bayesian inferences. They are readily available in most Bayesian computation libraries. Based on the recent improvements to the \(\hat{R}\) statistic (Vehtari et al. (2021)), it is recommended to use the samples only if \(\hat{R} < 1.01\) and \(\text{ESS} > 400\).
3.2.5 Step 3: model checking and validation
After model specification and learning, the third step of the workflow is model checking and validation. It should be conducted before any conclusions are drawn, and before the prediction accuracy of the model is estimated.
- The posterior predictive distribution
The basic way of checking the fit of a model to data is to draw simulated values from the trained model and compare them to the observed data. In a non-Bayesian framework, we would pick the most likely point estimate of parameters, such as the maximum likelihood estimate, use it to compute the model output \(\hat{y}\), and conduct residual analysis. In a Bayesian framework, posterior predictive checking allows some more possibilities.
The posterior predictive distribution is the distribution of the observable \(\tilde{y}\) (the model output) conditioned on the observed data \(y\): \[\begin{equation} p\left(\tilde{y}|y\right) = \int p\left(\tilde{y}|\theta\right) p\left(\theta | y\right) \mathrm{d}\theta \tag{3.14} \end{equation}\] This definition is very similar to the prior predictive distribution given in Sec. 3.2.3, except that the prior \(p(\theta)\) has been replaced by the posterior \(p(\theta|y)\). Similarly, it is simple to compute if the posterior has been approximated by an MCMC procedure: we first draw a finite number of parameter vectors \(\theta^{(m)}\) from the posterior distribution, and use each of them to compute a model output \(\tilde{y}^{(m)}\): \[\begin{align} \theta^{(m)} & \sim p(\theta|y) \tag{3.15}\\ \tilde{y}^{(m)} & \sim p(y|\theta^{(m)}) \tag{3.16} \end{align}\] This set of model outputs approximates the posterior probability distribution.
The following methods of model checking may apply to either a frequentist or a Bayesian framework.
If the fitting returns a point estimate of parameters \(\hat{\theta}\), then a single profile of model output \(\hat{y}\) can be calculated from it, either to be compared with the training data set \(y_\mathit{train}\) or to a separate test data set \(y_\mathit{test}\).
If the fitting returns a posterior distribution \(p(y|\theta)\), the same comparisons may be applied to any of the \(\tilde{y}^{(m)}\) samples.
Measures of model adequacy
After fitting, either ordinary linear regression or more sophisticated ones, some metrics may assess the predictive accuracy of the model.
- The R-squared (\(R^2\)) index is the proportion of the variance of the dependent variable that is explained by the regression model (closest to 1 is better)
\[\begin{equation} R^2 = 1-\frac{\sum_{i=1}^N\left(y_i - \hat{y}_i\right)^2}{\sum_{i=1}^N\left(y_i - \bar{y}_i\right)^2} \tag{3.17} \end{equation}\]
- The root-mean-square-error (RMSE) simply measures the differences between model predictions \(\hat{y}\) and observations \(y\) (lower is better)
\[\begin{equation} \mathrm{CV(RMSE)} = \frac{1}{\bar{y}} \sqrt{\frac{\sum_{i=1}^N\left(\hat{y}_i-y_i\right)^2}{N}} \tag{3.18} \end{equation}\]
- Coverage Width-based Criterion (CWC) (Chong, Augenbroe, and Yan (2021)), an indicator for probabilistic forecasts which measures the quality of the predictions based on both their accuracy and precision.
The \(R^2\) and CV-RMSE indices are too often treated as validation metrics. If they are calculated using a test data set, they can indeed estimate the model predictive ability outside of the training data set. They however do not ensure that the model correctly captures the data generating process: this is what residual analysis is for.
- Residual analysis
The hypothesis of an unbiased model assumes that the difference between the model output and the observed temperature is a sequence of independent, identically distributed variables following a Gaussian distribution with zero mean and constant covariance. In the example of a linear regression model, this condition may read: \[\begin{equation} r_i = y_i - \left( \hat{\theta}_0 + \hat{\theta}_1 X_{i,1} + \hat{\theta}_2 X_{i,2} \right) \sim N(0,\sigma) \tag{3.19} \end{equation}\] where \(r_i\) are the prediction residuals. Residual analysis is the process of checking the validity of their four hypotheses (independence, identical distribution, zero mean, constant variance), and the main step of model validation. It allows identifying problems that may arise after fitting a regression model (James et al. (2013)), among which: correlation of error terms, outliers, high leverage points, colinearity…
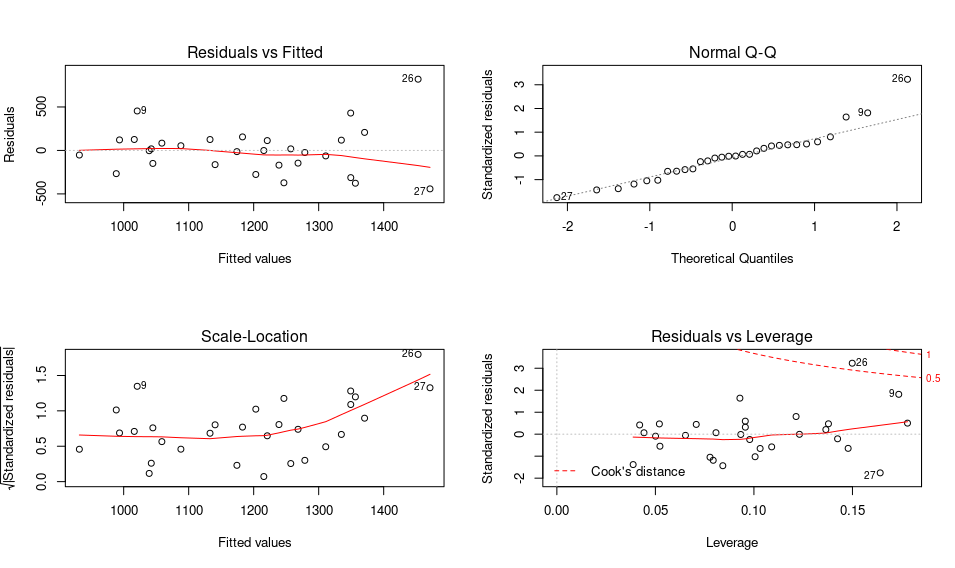
Figure 3.3: Example of residual plots after an ordinary linear regression in R: fitted vs residuals, Q-Q plot, scale location and residuals vs leverage
Residual analysis can be performed by an array of tests and graphs, some of which are shown on Fig. 3.3.
- A simple plot of the residuals versus the model output should not display any trend. The same goes for a plot of residuals vs any of the explanatory variables. Should a trend be visible, the model structure is probably insufficient to explain the data.
- A quantile-quantile (Q-Q) plot (upper right) is a way to check if the distribution of residuals is approximately Gaussian
- The scale-location plot (lower left) is a way to check the hypothesis of homoskedasticity, i.e. constant variance
- The residuals vs leverage plot (lower right) allows identifying eventual outliers and high leverage points.
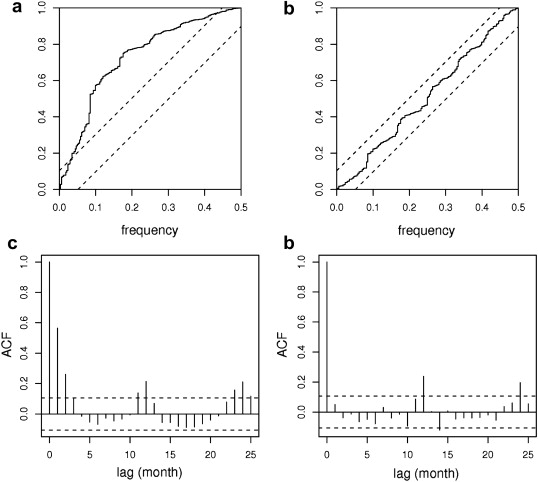
Figure 3.4: Autocorrelation function (top) and cumulated periodogram (bottom) of an insufficient model (left) and a sufficient model (right)
Most importantly, the correlation among the error terms should be checked. The autocorrelation function (ACF) checks the independence of residuals and may reveal lag dependencies which suggest influences that the model does not properly take into account. This is particularly important for time series models, and therefore well explained the time series litterature (Shumway and Stoffer (2000)). Alternatively, the Durbin-Watson test quantitatively checks for autocorrelation in regression models. “If there is correlation among the error terms, then the estimated standard errors will tend to underestimate to true standard errors. As a result, confidence and prediction intervals will be narrower than they should be.”(James et al. (2013))
If residuals display unequal variances or correlations, then the inferences and predictions of the fitted model should not be used. The model should be modified and re-trained according to the practitioner’s expertise and diagnostics of the analysis: additional explanatory variables can be included if possible.
- Posterior predictive checking
Posterior predictive checking uses global summaries to check the joint posterior predictive distribution \(p\left(\tilde{y}|y\right)\). Lack of fit of the data with respect to the posterior predictive distribution can be measured by the tail-area probability, or \(p\)-value, of a test quantity, and computed using posterior simulations of \((\theta, \tilde{y})\). The reader is referred to the book of Gelman et al for this Bayesian treatment of model checking.
3.3 Model assessment and selection
Once a model has passed the validation criteria, we may assume that its structure is sufficient to explain the main mechanics of the data generating process. However, this does not ensure that this model is the most appropriate one to draw inferences from, or to use for future predictions. While validation metrics impose a lower bound on the necessary model complexity, there are upper bounds to it imposed by: the practical identifiability of parameters; the risk of overfitting and wrong predictions.
3.3.1 Model selection workflows
The “single-model training and validation” workflow shown on Fig. 3.2 is embedded in a larger process for the selection of the appropriate model complexity and structure. Sec. 3.2.5 has shown the validation metrics that models should pass before their results are used. These conditions impose a lower bound on the acceptable model complexity. Then, the following sections will discuss that the model complexity should have an upper bound as well.
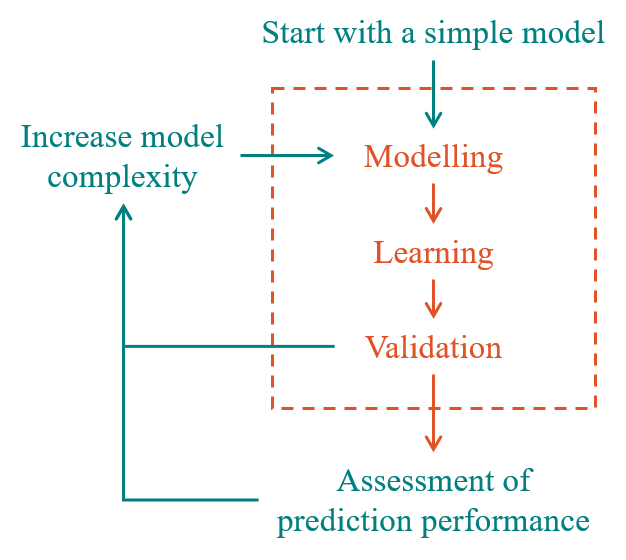
Figure 3.5: A workflow of gradually increasing model complexity: models of gradually increasing complexity must pass validation checks. The ones that do are compared in terms of predictive accuracy.
Two alternatives are shown on Fig. 3.5 and 3.6 for model selection among several possibilities of structures and complexities. The first one is the most common and intuitive: fitting models of gradually increasing complexity, keeping the ones that pass our validation checks, and comparing them in terms of predictive performance criteria. Inferences from simpler models may serve as starting values for more complex ones. This forward stepwise selection is suited to find a simple a robust model for prediction.
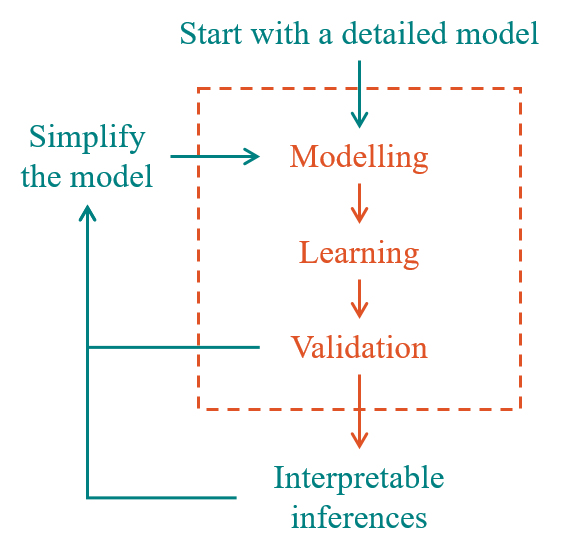
Figure 3.6: A workflow of gradually decreasing model complexity.
The second alternative, on Fig. 3.6, is backward selection: starting from a high number of predictors and fitting models of decreasing complexity. This approach is more suitable if the target is simply the estimation of parameter values: we try to find the upper bound of the information that can be learned from the data, and decrease our expectations in case of practical non-identifiability.
3.3.2 Sensitivity analysis
It is possible to filter out parameters that are unlikely to be learned from the data by running a preliminary sensitivity analysis. Parameter estimation algorithms are often computationally expensive and their cost quickly rises with the number of parameters of the model. This is a motivation for excluding parameters with little influence on the output, especially if we expect their posterior distributions to be close to their prior.
Sensitivity analysis is the main mathematical tool for the purpose of identifying the physical phenomena that can be really tested on the available experimental data. It measures the effects of parameter variations on the behaviour of a system and allows two things: ranking parameters by their significance so that non-influencial parameters may be filtered out, and identifying correlations between parameters which may prevent their estimation. Many local and global sensitivity analysis methods are applicable, providing first-order and total-order sensitivity indices from which correlations can be assessed: differential sensitivity analysis calculates the sensitivity of the model output to each parameter locally. Sampling-based methods (variance-based and one-at-a-time methods) allow a global sensitivity analysis but are more computationally intensive.
Variance-based methods (Iooss and Lemaître (2015)) decompose the total variance of the output into a sum of the partial variances representing the marginal effect of each input parameter independently. The sensitivity indices are the ratio of each variance to the total variance of the output. To calculate the indices, the variance of the output is obtained thorough sampling of the input space: although significantly costly, Monte-Carlo sampling methods enable to calculate first order and total indices. The state-of-the art RBD-FAST method (Goffart and Woloszyn (2021)) greatly mitigates the computational cost of variance-based methods. As it does not require a specific sampling scheme, it can be coupled to uncertainty analysis for no additional cost. RBD-FAST is one of the available methods in the SAlib python library.
3.3.3 Structural identifiability
Identifiability is the property of unicity and existence of a solution to an inverse problem.
The usual definition of identifiability originates from (Bellman and Åström (1970)). This notion was originally predominantly developed to help understanding complex biological systems, each of which is modelled by a specific set of differential equations with unobservable parameters. The question of identifiability is whether the input-output relation of the system may be explained by a unique parameter combination \(\theta\). \[\begin{equation} y(\theta) = y(\tilde{\theta}) \Rightarrow \theta = \tilde{\theta} \tag{3.20} \end{equation}\] Two conditions are required for the parameter estimates to be identifiable: the model structure must allow for parameters to be theoretically distinguishible from one another, with no redundancy; the data must be informative so that parameter uncertainty is not prohibitively high after identification. These conditions are respectively denoted structural and practical identifiability.
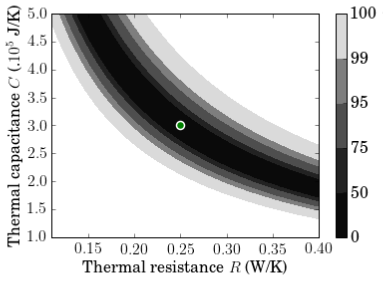
Figure 3.7: The RC thermal model of a house with no indoor heat input may be non-identifiable: only the product of resistance and capacitance is identifiable, resulting in infinitely many possible solutions for individual parameters.
Structural identifiability* is a purely mathematical property of the model structure and is an absolute necessary condition before applying data to a model. In a non-identifiable model, an infinity of posible parameter combinations may yield identical likelihoods. The Ph.D. thesis of Sarah Juricic (Juricic (2020)) reviewed methods for verifying structural identifiability of linear and non-linear systems, and proposed conditions for building thermal models to be identifiable.
Practical identifiability relates the parameter estimation possibilities to the experimental design (type and amount of measurements), the richness of available data and its accuracy, in addition to accounting for the type of model used. A parameter within a model is identifiable in practice if the data brings enough information to estimate it with finite confidence intervals (Rouchier (2018)). This property is related to sensitivity indices to some extent: a parameter which is deemed non-influencial by a sensitivity analysis has little impact on the model output variance, and has therefore little chance to be learned effectively from data. Sensitivity analysis is however not a sufficient condition for practical identifiability. Inference diagnostics, performed after learning, are the only way to check “how much information” the data has “brought to” each model parameter.
3.3.4 Practical identifiability
The main result we often expect from a fitted model is the value of its parameters, or some metrics computed from them. It is important to check whether all individual parameters are statistically significant, identify interactions between them, and assess how much the model has learned from data. Practical identifiability is a question of sufficiency of the data relatively to the model complexity.
- In a frequentist framework
Frequentist inference returns a point estimate of parameters \(\hat{\theta}\) and their covariance matrix \(\mathrm{cov}(\hat{\theta})\), assuming that the error is Gaussian. From this, statistical \(t\)-tests may be run to check for the significance of individual parameters, and point out strong correlations which would incite to reformulate the model.
However, confidence intervals obtained from \(\mathrm{cov}(\hat{\theta})\) are finite and may not point out practical non identifiability. Likelihood-based confidence intervals (Raue et al. (2009)) are a more informative way to display structural and practical non-identifiability. A comprehensive application of this theory in a building physics application was proposed recently by Deconinck and Roels (Deconinck and Roels (2017)) to measure the identifiability of parameters of several RC models describing the thermal characteristics of a building component.
- In a Bayesian framework
Bayesian inference returns a more complete description of the multivariate posterior distribution. This distribution is not only described by a mean and covariance matrix, but by a finite number of points which are not restricted to a multivariate Normal density.
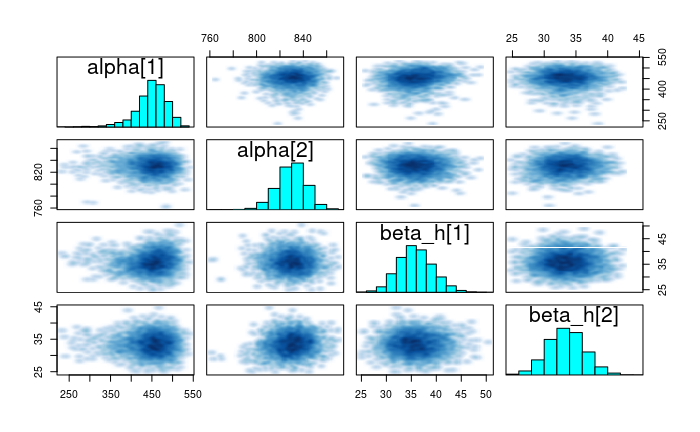
Figure 3.8: Pairplot of the multivariate posterior distribution approximated by MCMC, of a four-parameter model
Although a Bayesian analysis with proper prior knowledge is always feasible, if there is no learning from the data, i.e. from the likelihood, then the prior and posterior distributions will be identical. In this sense, identifiability in a Bayesian framework is close to the notion of identifiability in a frequentist approach as it is a matter of learning from the likelihood.
Considering \(\theta=(\theta_1, \theta_2)\) a set of parameters divided into two subsets, the subset \(\theta_2\) is not identified by the data if the observation does not increase our prior knowledge about \(\theta_2\) given \(\theta_1\): \[\begin{equation} p(\theta_2|\theta_1, y) \approx p(\theta_2 |\theta_1) \tag{3.21} \end{equation}\] To this purpose, Xie and Carlin(Xie and Carlin (2006)) propose a metric based on the Kullback-Leibler (KL) divergence, a quantity largely used for measuring the difference between two distributions. It measures how much is left to learn given data \(y\) and is defined as: \[\begin{equation} D_{\theta_1,y} = KL\left(p(\theta_2|\theta_1),p(\theta_2|y)\right) = \int_{-\infty}^{\infty}p(\theta_2|\theta_1) \mathrm{log}\frac{p(\theta_2|\theta_1)}{p(\theta_2|y)} \mathrm{d}\theta_2 \tag{3.22} \end{equation}\] This metric can be estimated with an MCMC approach, however requiring a second complete sampling of the posterior with the identifiable parameters fixed.
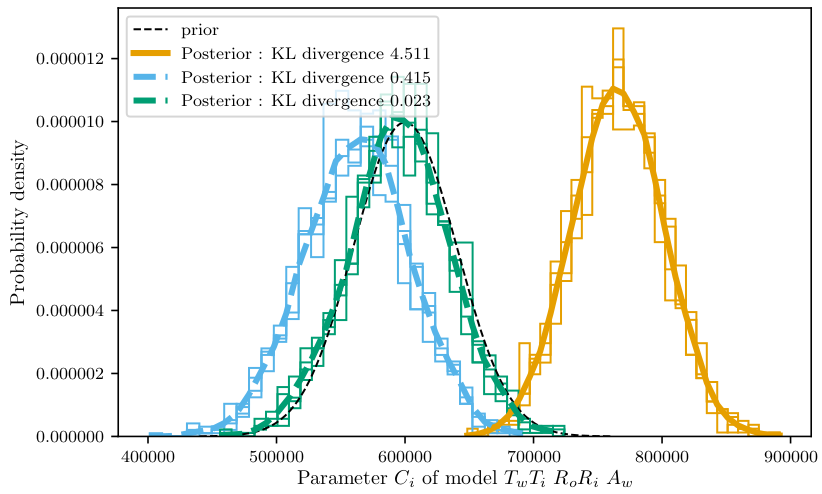
Figure 3.9: Illustration of the Kullback Leibler divergence for three posterior distributions. The less identifiable a parameter, the closer the posterior is to the prior, and the lower the KL divergence.
3.3.5 Model comparison criteria
A model should be complex enough to capture the data-generating process and pass the validation tests, but avoid overfitting. The statistical learning litterature abundantly warns readers against the risks of overfitting: a model with too many degrees of freedom will fit the training data very well, but will poorly extrapolate to new data, because it will reproduce specific patterns caused by local errors.
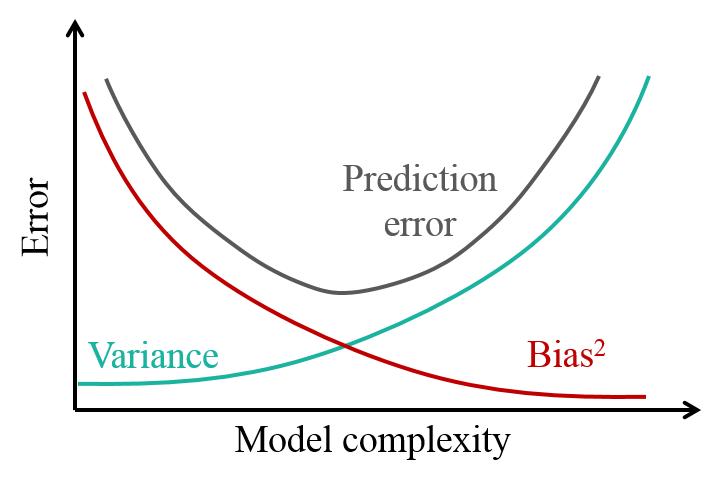
Figure 3.10: The bias-variance tradeoff
Fig. 3.10 illustrates the bias-variance tradeoff formulated in the ISL (James et al. (2013)): In order to minimize the expected test error, we need to select a statistical learning method that simultaneously achieves low variance and low bias. Variance refers to the amount by which \(\hat{y}\) would change if we estimated it using a different training data set. Bias refers to the error that is introduced by approximating a real-life problem.
Model selection criteria are designed to help comparing several models, not just based on their fit with training data, but on an estimation of their prediction accuracy with new data. These criteria often reward models that offer a good compromise between simplicity and accuracy. They include:
- The likelihood ratio method consists in assessing whether increasing the complexity of a model results in a significant improvement of likelihood which justifies this increased complexity (Bacher and Madsen (2011)). It is a computationally inexpensive method, since it only relies on the value of the total likelihood function, but can only apply to compare nested models.
- Cross-validation methods capture out-of-sample prediction error by fitting the model to training data and evaluating this predictive accuracy on a holdout set. They can be computationally expensive but avoid the problem of overfitting.
- Information criteria estimate the expected out-of-sample prediction error from an adjustment of the within-sample error (Gelman, Hwang, and Vehtari (2014)). A fully Bayesian criterion is the Widely Applicable Information Criterion (WAIC) (Watanabe and Opper (2010)), asymptotically equal to the Bayesian leave-one-out cross validation criterion.
The Pareto-smoothed importance sampling leave-one-out (PSIS-LOO) cross validation (Vehtari, Gelman, and Bagry (2016)) can be considered the state-of-the-art Bayesian criterion of model comparison and selection. The method does not require nested models, has a fast computation time and is asymptotically equivalent to WAIC.
The expected log pointwise predictive density for a new dataset (elpd) is a measure of predictive accuracy for the \(n\) data points of a given dataset, taken one at a time. The Bayesian LOO estimate of out-of-sample predictive fit is: \[\begin{equation} \mathrm{elpd}_\mathrm{loo} = \sum_{i=1}^n \mathrm{log}p(y_i|y_{-i}) \tag{3.23} \end{equation}\] where \[\begin{equation} p(y_i|y_{-i}) = \int p(y_i|\theta)p(\theta|y_{-i})\mathrm{d}\theta \tag{3.24} \end{equation}\] is the leave-one-out predictive density given the data without the \(i\)th data point.
Exact LOO cross-validation is costly, since it requires fitting the model as many times as there are observations, each time leaving out one observation. Instead, PSIS-LOO (Vehtari, Gelman, and Bagry (2016)) approximates the LOO estimate, using the pointwise log-likelihood values computed from samples of the posterior.
LOO cross-validation in general should be interpreted as a measure of prediction accuracy in the same conditions a model was trained. A better view of a model’s adaptability to new situations would likely be offered by a clear separation of the training and test datasets. Nevertheless, LOO is appropriate for model selection in several applications, including those of the present study: short-term prediction accuracy for model predictive control, and performance assessment of the building envelope.
The effective number of parameters \(p_\mathrm{eff}\) is another measure of the complexity of a model. It can be used to compare the complexity of a non-parametric model such as a Gaussian Process, with that of a parametric model, and is an additional tool for interpretation of a model selection procedure.